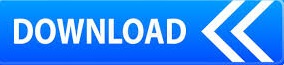
There is another slightly different version of the finite population correction in the literature which is given here.
n is the number of observations in the sample,.SE ( ) is the standard error of the sample mean,.If the sample size equals the population size, the standard error will be zero. If you look at the formula below, you will see that it reduces the standard error more and more as the sample size approaches the population size. Hence the need for a finite population correction. In the most extreme case, if your sample contained the entire population you would get the same value for the mean each time - in which case the standard error of the mean should be zero. Clearly there will not be much variability between sample means because the samples will mostly contain the same individuals. Imagine you take several samples each comprising nearly all members of a population. But if your sample comprises a large part of the population, the usual equation for the standard error will over-estimate the standard error. The formulae given above for estimating the standard error assume you are taking a sample from an infinite population.
So, if you intend to quote standard errors, and your sample sizes are small, you should use the corrected standard deviation in the formula. We noted previously that the sample standard deviation (s) is a biased estimator of the population standard deviation (σ).
the standard error of the mean is used to estimate the variation in a set of means. the standard deviation of the observations is used to describe the variation in a set of observations. The standard deviation of the observations and the standard error of the mean are frequently confused. The larger the number of observations, the smaller will be the standard error. The larger the variability, the greater will be the standard error. Hence the magnitude of the standard error of the mean depends on both the variability of the observations (s) and the number of observations (n). n is the number of observations in the sample. In practice we obtain an unbiased estimate of the standard error of a mean by dividing the sample standard deviation (s) by the square root of the number of observations in that sample. It is equal to the population standard deviation (σ) divided by the square root of the number of observations in that sample. The standard error of the mean (SE ) is somewhat unusual in that there is a simple algebraic formula for it - and the formula is valid irrespective of the distribution of the data. As such it is an inferential statistic rather than a descriptive statistic. Hence, unlike the standard deviation of the observations, the standard error of the mean is estimated rather than measured. Usually of course we only calculate one mean for a set of data, not multiple means. Thus the terms 'standard error of the mean', 'standard deviation of the mean' and 'standard error' may all mean exactly the same thing! The term standard error of the mean is commonly (though imprecisely) shortened to just standard error. In other words it is the standard deviation of a large number of sample means of the same sample size drawn from the same population. The standard error of the mean is the standard deviation of the sampling distribution of the mean.